
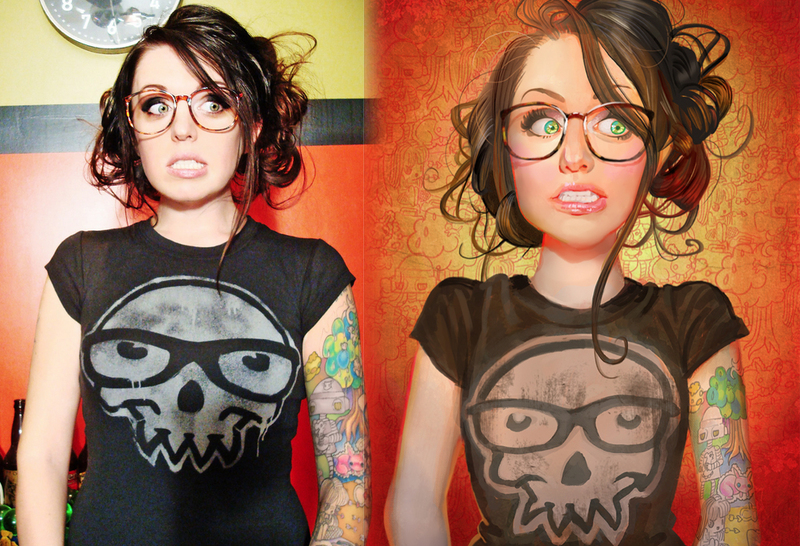
However, these methods work only with a single style applied during training. In order to reduce the computational complexity, several feed-forward CNN-based methods have been proposed ulyanov2016texture ulyanov2016instance johnson2016perceptual dumoulin2016 li2017diversified chen2016fast zhang2017multi huang2017arbitrary. Since their method generates the stylized images by iteratively updating the feature maps of CNNs, it is computationally costly. gatys2016controlling achieve promising results by transferring different styles of artworks to images via the semantic contents space. With the use of Convolutional Neural Networks (CNN), Gatys et al. While recovering photorealistic images from portraits is still uncommon in the literature, image stylization methods have been widely studied. Therefore, landmark detectors often fail to localize facial landmarks accurately as shown in Figure 1(c). In general, stylized face images contain a variety of facial expressions, facial feature distortions and misalignments. Since facial details and expressions in stylized portraits often undergo severe distortions and become corrupted by artifacts such as profile edges and color changes e.g., as in Figure 1(b), recovering a photorealistic face image from its stylized counterpart is very challenging. Latent photorealistic face images recovered from their artistic portraits are interpretable for humans and they may be useful in facial analysis. Unlike prior research on the image stylization, we address a challenging inverse problem of photorealistic face recovery from stylized portraits which aims at recovering a photorealistic image of face from a given stylized portrait. Style transferring methods are powerful tools that can generate portraits in various artistic styles from photorealistic images. Portraits, artistic paintings, and hand-drawn sketches.
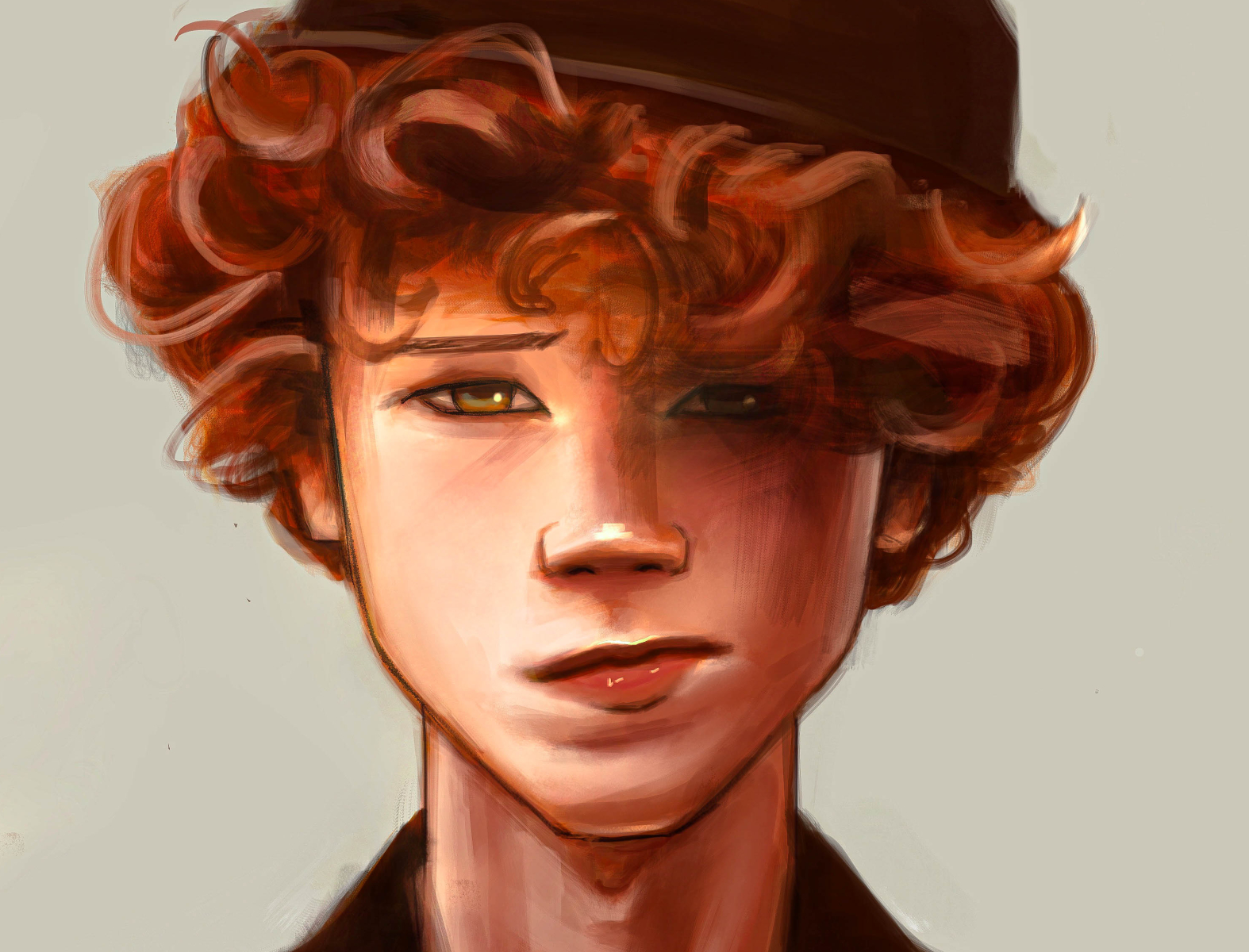
InĪddition, our method can recover photorealistic faces from unseen stylized Method achieves superior face recovery and attains state-of-the-art results. Synthesized dataset and a hand-drawn sketch dataset, we demonstrate that our By conducting extensive evaluations on a large-scale Photorealistic faces from unaligned portraits while preserving the identity of DN has multipleĬonvolutional and fully-connected layers, and its role is to enforce recoveredįaces to be similar to authentic faces. Via a distance measure which compares features of recovered and ground truthįaces extracted from a pre-trained FaceNet network. Promote the recovered and ground truth faces to share similar visual features (STN), SRN automatically compensates for misalignments of stylized portraits to Our SRN,Ĭomposed of an autoencoder with residual block-embedded skip connections, isĭesigned to transfer feature maps of stylized images to the feature maps of theĬorresponding photorealistic faces. Style Removal network (SRN) and a Discriminative Network (DN). Identity-preserving Face Recovery from Portraits (IFRP) method that utilizes a Often distorted or fully lost in artistic portraits. Preserves the subject's identity is challenging because the facial details are
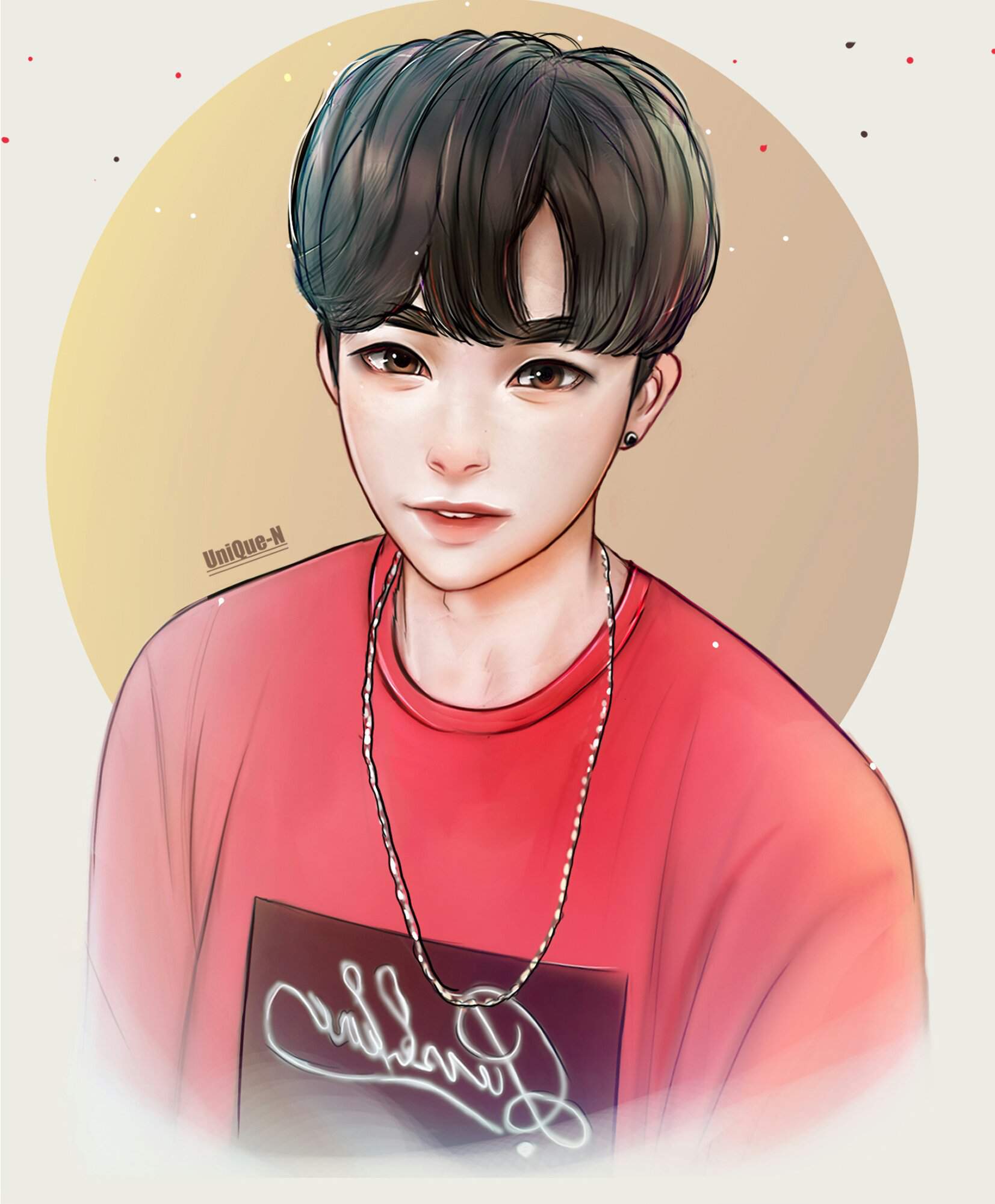
Given an artistic portrait, recovering the latent photorealistic face that
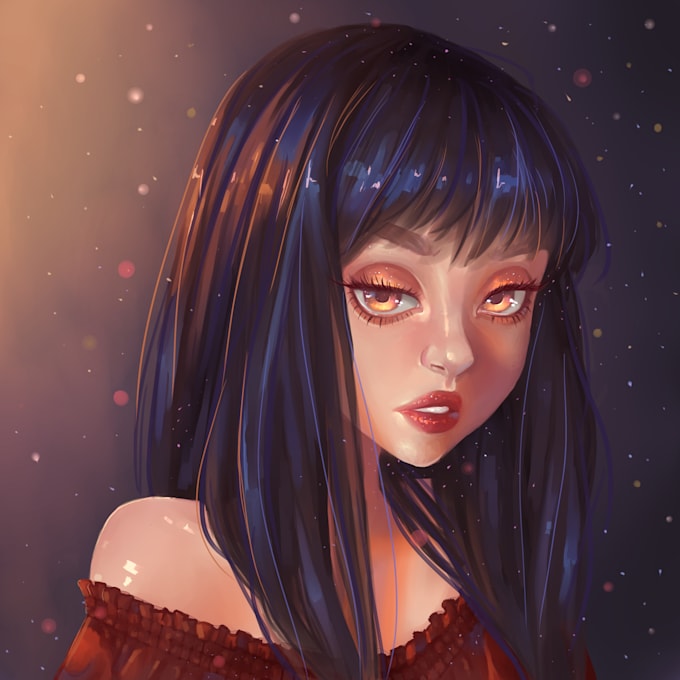
Identity-preserving Face Recovery from Stylized Portraits
